Heterogeneous treatment effects via two-stage DID
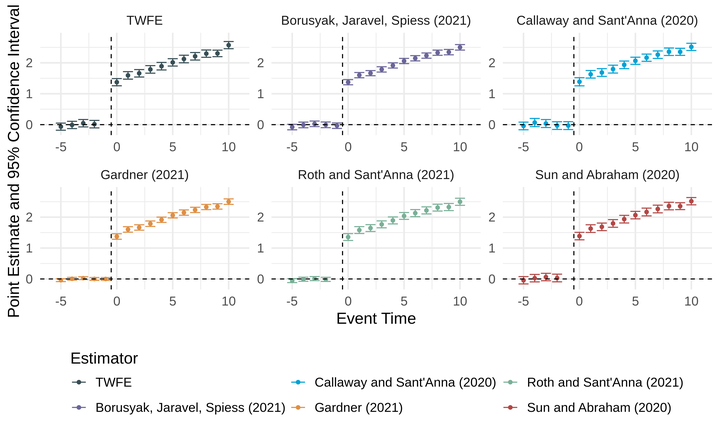
Homogeneous Treatment Effects
🎯 Purpose: Estimate treatment effects when the treatment is not randomly assigned.
📉 Parallel Trends Assumption: In the absence of treatment, the treated and untreated groups would have followed parallel paths over time.
🔄 Two-Way Fixed-Effects (TWFE) Model:
- Static Model:
$$ y_{igt} = \mu_g + \eta_t + \tau D_{gt} + \epsilon_{igt} $$
- $ y_{igt} $: Outcome variable.
- $ i $: Individual.
- $ t $: Time.
- $ g $: Group.
- $ \mu_g $: Group fixed-effects.
- $ \eta_t $: Time fixed-effects.
- $ D_{gt} $: Indicator for treatment status.
- $ \tau $: Average treatment effect on the treated (ATT).
❗ Limitations: Assumes constant treatment effects across groups and time, which is often unrealistic.
Heterogeneous Treatment Effects
- 🔄 Enhanced TWFE Model:
$$
y_{igt} = \mu_g + \eta_t + \tau_{gt} D_{gt} + \epsilon_{igt}
$$
- Allows treatment effects ($ \tau_{gt} $) to vary by group and time.
- Aggregates group-time average treatment effects into an overall average treatment effect ($ \tau $).
Dynamic Event-Study TWFE Model
🔄 Model: $$ y_{igt} = \mu_g + \eta_t + \sum_{k=-L}^{-2} \tau_k D_{gt}^k + \sum_{k=0}^{K} \tau_k D_{gt}^k + \epsilon_{igt} $$
- Allows for treatment effects to change over time.
- $ D_{gt}^k $: Lags and leads of treatment status.
- Coefficients ($ \tau_k $) represent the average effect of being treated for $ k $ periods.
🎯 Estimation Goals:
- Objective: Estimate the average treatment effect of being exposed for $ k $ periods.
- Average Treatment Effect:
$$
\tau_k = \sum_{g,t : t-g=k} \frac{N_{gt}}{N_k} \tau_{gt}
$$
- $ N_{gt} $: Number of observations in group $ g $ and time $ t $.
- $ N_k $: Total number of observations with $ t - g = k $.
Negative Weighting Problem
- ❗ Issue: Traditional TWFE models can produce estimates with negative weights, leading to biased overall treatment effect estimates.
- 🛠 Solution by Gardner (2021):
- Use a two-stage approach to estimate group and time fixed-effects from untreated/not-yet-treated observations and then estimate treatment effects using residualized outcomes.
Two-stage differences in differences
🌱 Gardner (2021) Approach:
🔍 Key Insight: Under parallel trends, group and time effects are identified from the untreated/not-yet-treated observations.
📜 Procedure:
🥇 First Stage:
Estimate the model:
\begin{equation} y_{igt} = \mu_g + \eta_t + \epsilon_{igt} \end{equation}
Using only untreated/not-yet-treated observations ($D_{gt} = 0$).
Obtain estimates for group and time effects ($\mu_g$ and $\eta_t$).
🥈 Second Stage:
- Regress adjusted outcomes ($y_{igt} - \mu_g - \eta_t$) on treatment status ($D_{gt}$) in the full sample to estimate treatment effects ($\tau$).
🎯 Rationale:
- The parallel trends assumption implies that residuals ($\epsilon_{igt}$) are uncorrelated with the treatment dummy, leading to a consistent estimator for the average treatment effect.